Keynote Lectures
Anastasia Ailamaki, Professor and Lab Director, Data-Intensive Applications and Systems Laboratory, École Polytechnique Fédérale de Lausanne, Switzerland
Fast, just-in-time queries on heterogeneous scientific data
Efthimios Kaxiras, John Hasbrouck Van Vleck Professor of Pure and Applied Physics, Harvard University, USA
Machine Learning for the Materials World
Michael Norman, Director of the San Diego Supercomputer Center, UC San Diego, USA
The Assembly of the First Galaxies: 20 Years of Computational Progress
Tomaso Poggio, Eugene McDermott Professor, MIT, USA
Why and When Can Deep – but Not Shallow – Networks Avoid the Curse of Dimensionality: Theoretical Results
Olga Sorkine-Hornung, Professor ETH Zurich, Switzerland
Interactive 3D Modeling and Digital Fabrication using Computation-Friendly Variational Methods
Rick L. Stevens, Associate Laboratory Director for Computing, Environment and Life Sciences, Argonne National Laboratory, USA
Deep Learning In Cancer And Infectious Disease: Novel Driver Problems For Future HPC Architecture
Stefan Thurner, Head of the Section for Science of Complex Systems, Medical University of Vienna, Austria
Big-Data driven 1-to-1 Simulations of Financial Systems for the Elimination of Systemic Risk
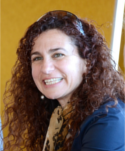
Professor and Lab Director, Data-Intensive Applications and Systems Laboratory, École Polytechnique Fédérale de Lausanne, Switzerland
WEB
Anastasia Ailamaki is a Professor of Computer and Communication Sciences at the Ecole Polytechnique Federale de Lausanne (EPFL) in Switzerland. Her research interests are in data-intensive systems and applications, and in particular (a) in strengthening the interaction between the database software and emerging hardware and I/O devices, and (b) in automating data management to support computationally- demanding, data-intensive scientific applications. She has received an ERC Consolidator Award (2013), a Finmeccanica endowed chair from the Computer Science Department at Carnegie Mellon (2007), a European Young Investigator Award from the European Science Foundation (2007), an Alfred P. Sloan Research Fellowship (2005), eight best-paper awards in database, storage, and computer architecture conferences, and an NSF CAREER award (2002). She holds a Ph.D. in Computer Science from the University of Wisconsin-Madison in 2000. She is an ACM fellow and the vice chair of the ACM SIGMOD community, a senior member of the IEEE, and an elected member of the Swiss National Research Council. She has served as a CRA-W mentor, is a member of the Expert Network of the World Economic Forum.
Today’s scientific processes heavily depend on fast and accurate data analysis. Scientists are routinely overwhelmed by the effort needed to manage the volumes of data produced either by observing phenomena or by sophisticated simulations. As data management software is often inefficient, hard to manage, or too generic to serve scientific applications, the scientific community typically uses special-purpose legacy software. With the exponential growth of dataset size and complexity, however, application-specific systems no longer scale to efficiently analyse the relevant parts of their data, thereby slowing down the cycle of analysing, understanding, and preparing new experiments. I will illustrate the different nature of problems we faced when managing brain simulation and patient data for neuroscience applications, and will show how the problems from neuroscience translate into challenges for the data management community. These challenges inspire new technologies which overturn long-stangding assumptions, enable meaningful, timely results and advance scientific discovery. Finally I will describe the challenges associated with gaining access to medical neuroscience data and using it toward advancing our understanding of the functionality of the brain.
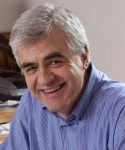
John Hasbrouck Van Vleck Professor of Pure and Applied Physics, Harvard University, USA
WEB
Efthimios Kaxiras was educated at the Massachusetts Institute of Technology where he received a PhD in theoretical condensed matter physics. He joined the faculty of Harvard University in 1991, where he is currently the John Hasbrouck Van Vleck Professor of Pure and Applied Physics in the Department of Physics and the School of Engineering and Applied Sciences. He is the Founding Director of the Institute for Applied Computational Science and served as the Director of the Initiative on Innovative Computing. He has also served in faculty appointments and in administrative positions in Switzerland (EPFL) and in Greece (University of Crete, University of Ioannina, FoRTH). He holds several distinctions, including Fellow of the American Physical Society and Chartered Physicist and Fellow of the Institute of Physics (London).
His research interests encompass a wide range of topics in the physics of solids and fluids, with recent emphasis on materials for renewable energy, especially batteries and photovoltaics, and on simulations of blood flow in coronary arteries. He serves on the Editorial Board of several scientific journals, has published over 360 papers in refereed journals and several review articles and chapters in books, as well as a graduate textbook on the properties of solids. His group has developed several original methods for efficient simulations of solids using high-performance computing as well as multiscale approaches for the realistic modeling of materials.
The last few years have witnessed a surge of activity in machine learning approaches applied to materials science. In this talk I will address both the promise and the limitations of using data science ideas to explore the possibilities of “materials by design”, drawing on examples from recent research in our group. Applications of our work focus on exploring the properties of new materials for energy related problems, including improved batteries, photovoltaics, and new catalysts; in a parallel but distinct type of approach, we have been exploring how machine learning approaches can shed light into fundamental questions like the strength of amorphous solids.
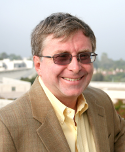
Director of the San Diego Supercomputer Center, UC San Diego, USA
WEB
Dr. Michael L. Norman, named SDSC interim director in June 2009 and appointed to the position of director in September 2010, is a distinguished professor of physics at UC San Diego and a globally recognized astrophysicist. Dr. Norman is a pioneer in using advanced computational methods to explore the universe and its beginnings. In this capacity, he has directed the Laboratory for Computational Astrophysics — a collaborative effort between UC San Diego and SDSC resulting in the Enzo community code for astrophysics and cosmology in use worldwide.
Dr. Norman is the author of over 300 research articles in diverse areas of astrophysics, including star and galaxy formation, the evolution of intergalactic medium, as well as numerical methods. Dr. Norman’s work has earned him numerous honors, including Germany’s prestigious Alexander von Humboldt Research Prize, the IEEE Sidney Fernbach Award, and several HPCC Challenge Awards. He also is a Fellow of the American Academy of Arts and Sciences, and the American Physical Society. He holds an M.S. and Ph.D. in engineering and applied sciences from UC Davis, and in 1984 completed his post-doctoral work at the Max Planck Institute for Astrophysics in Garching, Germany. From 1986 to 2000, Dr. Norman held numerous positions at the University of Illinois in Urbana, as an NCSA associate director and senior research scientist under Larry Smarr, and as a professor of astronomy. From 1984 to 1986, he was a staff member at Los Alamos National Laboratory.
Dr. Norman is the Principal Investigator of two of SDSC’s leading HPC systems—Gordon and Comet—which together represent more than $42M in NSF funding.
In this talk I give a progress report on my attempts to reconstruct the first billion years of cosmic evolution beginning with the formation of the first generation of stars and culminating in the complete photoionization of the intergalactic medium. After 20 years of intense effort, the narrative is falling into place. Progress has been achieved through the development and application of complex multiphysics numerical simulations of increasing physical complexity and scale on the most powerful supercomputers. I describe the processes that govern the formation of the first generation of stars, the transition to the second generation of stars, the assembly of the first galaxies, and finally the reionization of the universe. I discuss the observational predictions of these simulations which will be tested with the next generation observatories, principally the James Webb Space Telescope to be launched into space ca.2018.

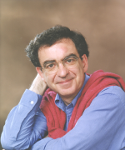
Eugene McDermott Professor, MIT, USA
WEB 1 & WEB 2
Tomaso A. Poggio, is the Eugene McDermott Professor in the Dept. of Brain & Cognitive Sciences at MIT and the director of the new NSF Center for Brains, Minds and Machines at MIT of which MIT and Harvard are the main member Institutions. He is a member of both the Computer Science and Artificial Intelligence Laboratory and of the McGovern Brain Institute. He is an honorary member of the Neuroscience Research Program, a member of the American Academy of Arts and Sciences, a Founding Fellow of AAAI and a founding member of the McGovern Institute for Brain Research. Among other honors he received the Laurea Honoris Causa from the University of Pavia for the Volta Bicentennial, the 2003 Gabor Award, the Okawa Prize 2009, the AAAS Fellowship and the 2014 Swartz Prize for Theoretical and Computational Neuroscience. He is one of the most cited computational scientists with contributions ranging from the biophysical and behavioral studies of the visual system to the computational analyses of vision and learning in humans and machines. With W. Reichardt he characterized quantitatively the visuo-motor control system in the fly. With D. Marr, he introduced the seminal idea of levels of analysis in computational neuroscience. He introduced regularization as a mathematical framework to approach the ill-posed problems of vision and the key problem of learning from data. The citation for the 2009 Okawa prize mentions his “…outstanding contributions to the establishment of computational neuroscience, and pioneering researches ranging from the biophysical and behavioral studies of the visual system to the computational analysis of vision and learning in humans and machines.” His research has always been interdisciplinary, between brains and computers. It is now focused on the mathematics of deep learning and on the computational neuroscience of the visual cortex. A former Corporate Fellow of Thinking Machines Corporation and a former director of PHZ Capital Partners, Inc., is a director of Mobileye and was involved in starting, or investing in, several other high tech companies including Arris Pharmaceutical, nFX, Imagen, Digital Persona and Deep Mind. Among his PhD students and post-docs are some of the today’s leaders in the Science and in the Engineering of Intelligence, from Christof Koch (President and Chief Scientific Officer, Allen Institute) to Amnon Shashua (CTO and founder, Mobileye) and Demis Hassabis (CEO and founder, Deep Mind).
In recent years, by exploiting machine learning — in which computers learn to perform tasks from sets of training examples — artificial-intelligence researchers have built impressive systems. Two of my former postdocs — Demis Hassabis and Amnon Shashua — are behind the two main success stories of AI so far: AlphaGo bettering the best human players at Go and Mobileye leading the whole automotive industry towards vision-based autonomous driving. There is, however, little in terms of a theory explaining why deep networks work so well. In this talk I will review an emerging body of theoretical results on deep learning including the conditions under which it can be exponentially better than shallow learning. A class of deep convolutional networks represent an important special case of these conditions, though weight sharing is not the main reason for their exponential advantage. II will discuss implications of a few key theorems, together with open problems and conjectures.
I will also sketch the vision of the NSF-funded, MIT-based Center for Brains, Minds and Machines which strives to make progress on the science of intelligence by combining machine learning and computer science with neuroscience and cognitive science.
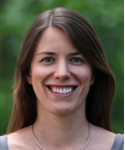
Professor, ETH Zürich, Switzerland
WEB
Olga Sorkine-Hornung is an Associate Professor of Computer Science at ETH Zurich, where she leads the Interactive Geometry Lab and is currently the director of the Institute of Visual Computing. Prior to joining ETH she was an Assistant Professor at the Courant Institute of Mathematical Sciences, New York University (2008-2011). She earned her BSc in Mathematics and Computer Science and PhD in Computer Science from Tel Aviv University (2000, 2006). Following her studies, she received the Alexander von Humboldt Foundation Fellowship and spent two years as a postdoc at the Technical University of Berlin. Olga is interested in theoretical foundations and practical algorithms for digital content creation tasks, such as shape representation and editing, modeling techniques, digital fabrication, computer animation and digital image manipulation. She also works on fundamental problems in digital geometry processing, including reconstruction, parameterization, filtering and compression of geometric data. Olga received the EUROGRAPHICS Young Researcher Award (2008), the ACM SIGGRAPH Significant New Researcher Award (2011), the ERC Starting Grant (2012), the ETH Latsis Prize (2012), the Intel Early Career Faculty Award (2013) and the EUROGRAPHICS Outstanding Technical Contributions Award (2017), as well as a number of Best Paper and Software awards. She was named Fellow of the Eurographics Association in 2015.
Digital 3D shapes are ubiquitously used in product design and engineering, architecture, simulator training, medicine and prosthetics, virtual and augmented reality, entertainment and art. With the advancement and democratization of modern fabrication technologies such as 3D printing and personal robotic fabrication, interactive and intuitive tools for geometric modeling and processing gain importance and spread. In this talk, I will discuss the research efforts of my lab in this domain, in particular in light of the growing resolution and proliferation of available geometric and visual data. I will focus on modeling with irregular polygonal meshes, since they are a powerful digital shape representation: such meshes are flexible and can represent virtually any complex shape; they are efficiently rendered by graphics hardware; they are the standard output of 3D acquisition and routinely used as input to simulation software. Yet irregular meshes are difficult to interactively model and edit because they lack a higher-level control mechanism. I will survey a series of research results on surface modeling via mesh deformation and show how high-resolution meshes can be interactively manipulated and animated in a real-time and intuitive manner. I will also discuss how the incorporation of some simple physics laws directly into the interactive modeling framework can be done inexpensively and beneficially for geometric modeling: while not being as restrictive and parameter-heavy as a full-blown physical simulation, this allows to creatively model shapes with improved realism and directly use them in fabrication.
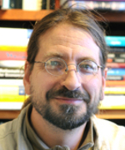
The Associate Laboratory Director for Computing, Environment and Life Sciences, Argonne National Laboratory, USA
WEB
Since 1999, Rick Stevens has been a professor at the University of Chicago and since 2004, an Associate Laboratory Director at Argonne National Laboratory. He is internationally known for work in high-performance computing, collaboration and visualization technology, and for building computational tools and web infrastructures to support large-scale genome and metagenome analysis for basic science and infectious disease research. He teaches and supervises students in the areas of computer systems and computational biology. He co-leads the DOE national laboratory group that has been developing the national initiative for Exascale computing.
Stevens is principle investigator for the NIH/NIAID supported PATRIC Bioinformatics Resource Center which is developing comparative analysis tools for infectious disease research and serves a large user community. Stevens is also the PI of The Exascale DeepLearning and Simulation Enabled Precision Medicine for Cancer project through the Exascale Computing Project (ECP), which focuses on building a scalable deep neural network code called the CANcer Distributed Learning Environment (CANDLE) to address three top challenges of the National Cancer Institute. Stevens is also one of the PIs for the DOE-NCI Joint Design of Advanced Computing Solutions for Cancer project, part of the Cancer Moonshot initiative. In this role, he leads a pilot project on pre-clinical screening aimed at building machine learning models for cancer drug response that will integrate data from cell line screens and patient derived xenograft models to improve the range of therapies available to patients.
Over the past twenty years, he and his colleagues have developed the SEED, RAST, MG-RAST and ModelSEED genome analysis and bacterial modeling servers that have been used by tens of thousands of users to annotate and analyze more than 250,000 microbial genomes and metagenomic samples.
At Argonne, Stevens leads the Computing, Environment and Life Sciences (CELS) Directorate that operates one of the top supercomputers in the world (a 10 Petaflops/s machine called MIRA). Prior to that role, he led the Mathematics and Computer Science Division for ten years and the Physical Sciences Directorate. He and his group have won R+D100 awards for developing advanced collaboration technology (Access Grid). He has published over 200 papers and book chapters and holds several patents. He lectures widely on the opportunities for large-scale computing to impact biological science.
The adoption of machine learning is proving to be an amazingly successful strategy in improving predictive models for cancer and infectious disease. In this talk I will discuss two projects my group is working on to advance biomedical research through the use of machine learning and HPC. In cancer, machine learning and in deep learning in particular, is used to advance our ability to diagnosis and classify tumors. Recently demonstrated automated systems are routinely out performing human expertise. Deep learning is also being used to predict patient response to cancer treatments and to screen for new anti-cancer compounds. In basic cancer research its being use to supervise large-scale multi-resolution molecular dynamics simulations used to explore cancer gene signaling pathways. In public health it’s being used to interpret millions of medical records to identify optimal treatment strategies. In infectious disease research machine learning methods are being used to predict antibiotic resistance and to identify novel antibiotic resistance mechanisms that might be present. More generally machine learning is emerging as a general tool to augment and extend mechanistic models in biology and many other fields. It’s becoming an important component of scientific workloads. From a computational architecture standpoint, deep neural network (DNN) based scientific applications have some unique requirements. They require high compute density to support matrix-matrix and matrix-vector operations, but they rarely require 64bit or even 32bits of precision, thus architects are creating new instructions and new design points to accelerate training. Most current DNNs rely on dense fully connected networks and convolutional networks and thus are reasonably matched to current HPC accelerators. However future DNNs may rely less on dense communication patterns. Like simulation codes power efficient DNNs require high-bandwidth memory be physically close to arithmetic units to reduce costs of data motion and a high-bandwidth communication fabric between (perhaps modest scale) groups of processors to support network model parallelism. DNNs in general do not have good strong scaling behavior, so to fully exploit large-scale parallelism they rely on a combination of model, data and search parallelism. Deep learning problems also require large-quantities of training data to be made available or generated at each node, thus providing opportunities for NVRAM. Discovering optimal deep learning models often involves a large-scale search of hyperparameters. It’s not uncommon to search a space of tens of thousands of model configurations. Naïve searches are outperformed by various intelligent searching strategies, including new approaches that use generative neural networks to manage the search space. HPC architectures that can support these large-scale intelligent search methods as well as efficient model training are needed.
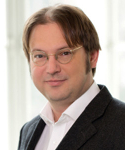
Head of the Section for Science of Complex Systems, Medical University of Vienna, Austria
WEB
Stefan Thurner has a background in both theoretical physics from the Technical University of Vienna and in economics from the University of Vienna before joining the faculty at the Medical University of Vienna.Thurner has published more than 190 scientific articles in fundamental physics (topological excitations in quantum field theories, entropy for complex systems), applied mathematics (wavelet statistics, fractal harmonic analysis, anomalous diffusion), complex systems (network theory, evolutionary systems), life sciences (network medicine, gene regulatory networks, bioinformatics, heart beat dynamics, cell motility), economics (price formation, regulation, systemic risk) and lately in social sciences (opinion formation, bureaucratic inefficiency, collective human behavior in virtual worlds). He holds 2 patents. His work has received broad interest from the media such as the New York Times, BBC world, Nature, New Scientist, Physics World and is featured in more than 400 newspaper, radio and television reports.
Controlling complex systems is a challenge that is as old as humanity. Unlike physical systems, which often can be described with a few parameters, complex systems usually depend on many details, often millions. For the first time, with the advent of a new data generation, we are in the position to measure all such details in real time. This opens completely new abilities for modeling, understanding and evemtually managing complex systems. We will present a 1:1 model of the financial market of an entire nation and show the knowledge of all transactions can help to eliminate the financial systemic risk of the country. We show that the systemic risk level of every agent in the system can be measured by simple network measures. With actual central bank data for Austria and Mexico we are able to compute the expected systemic losses of an economy, a number that allows us to estimate the cost of a financial crises. We can further show that it is even possible to compute the systemic risk of every single financial transaction. We suggest an intelligent financial transaction tax that taxes the systemic risk contribution of all transactions. With the 1:1 agent based model we demonstrate that this Systemic Risk Tax practically eliminates the network-component of systemic risk in a system.