ICCS is well known for its excellent line-up of keynote speakers.
This page will be frequently updated with new names, lecture titles and abstracts.
Robert Axtell, George Mason University, United States of America
Modeling Economies at Full-Scale: Every Worker, Every Firm
Peter Coveney, University College London, United Kingdom
Big AI: Blending Artificial Intelligence and Physics-based Approaches to Build Virtual Humans
Thomas Engels, Universität Rostock & Technische Universität Berlin, Germany
The Computational Planet – with Computational Insects: Wavelet-Based Adaptive Simulations of Flapping Flight
Neil Ferguson, Imperial College London, United Kingdom
Modelling and Simulation to Inform Policy Responses to Global Threats: Lessons from COVID-19
Giulia Galli, The University of Chicago, United States of America
Quantum Simulations of Materials and Molecules on Hybrid Quantum-Classical Architectures
Rebecca Wade, Heidelberg Institute for Theoretical Studies, Germany
Computational Challenges for Biomolecular Simulation Approaches to Drug Discovery
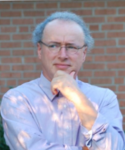
George Mason University, United States of America
WEB
I work at the intersection of the computational, social, behavioral and economic sciences. My research group combines agent-based computing with micro-data to build large-scale models having high verisimilitude with the real-world. We have worked on a variety of policy issues, from housing to fisheries, behavioral aspects of retirement and science policy. I supervise Ph.D. students in Mason’s Computational Social Science Ph.D. program. I also serve on Ph.D. committees from other departments when the work involves agent-based modeling, e.g., Economics, Systems Engineering and Operations Research, Public Policy, and Civil and Infrastructure Engineering. I have been visiting Professor at the University of Oxford (UK), the New School for Social Research (NY), and Middlebury College (Vermont). My research has been published in leading general interest journals (“Science,” “Nature” and “Proceedings of the National Academy of Sciences”), in field journals (e.g., “American Economic Review,” “Economic Journal”, “Computational and Mathematical Organization Theory,” “Journal of Industrial Ecology”), and reprised in newspapers (e.g., “Wall St. Journal,” “Washington Post”) and technology publications (e.g., “Scientific American,” “Technology Review,” “Wired”).
The growing availability of micro-level data has made it possible to construct high-fidelity models of economic processes at full-scale with actual economies. In this talk I will describe such a model of the U.S. private sector, involving some 120 million workers employed by nearly 6 million firms. A large number of gross patterns and regularities exist in the micro-data on firms and workers and in this talk I will describe how my group has built a large-scale agent-based model (ABM) that reproduces a substantial number of these features present in the data. An important part of creating such a model has been efficient parallelization and I will describe both what works and what does not work for parallelizing ABMs. Specifically, the importance of randomization and asynchronous agent activation for the suppression of computational artifacts will be emphasized. This model is described at length in my forthcoming book, “Dynamics of Firms: Data, Theories, and Models”.
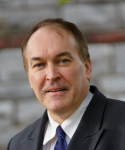
University College London, United Kingdom
WEB
In my position as Director of the Centre for Computational Science (CCS) and the Computational Life and Medical Sciences Network (CLMS) I lead a strong and sustained effort to enable cutting edge compute- and data-intensive scientific research on HPC and e-Science infrastructures worldwide. Within the CCS we tackle a wide range of scientific problems , from the life sciences to engineering, through simulation, thereby intensively using infrastructures such as the NGS, EGI, TeraGrid, DEISA and PRACE. Our investigations span time and length-scales from the macro-, through the meso- and to the nano- and microscales. We also embrace grid computing as a means to push our research beyond the boundaries of what can be achieved using a single computational resource, often performing single simulations that span multiple grid machines, and invoke tools such as computational steering and high performance visualisation.
We perform this research not in isolation, but as part of numerous large UK, EU and international collaborative projects (such as Genius, VPH-NoE, Virolab and MAPPER, but also the two recently awarded EU projects VECMA and CompBioMed2). In addition I am a founding editor of the new Journal of Computational Science, have published more than 400 scientific papers, edited 20 books and coauthored two best-selling popular science books (The Arrow of Time and Frontiers of Complexity, both with Roger Highfield).
This talk will introduce a productive blend of AI and physics-based (PB) approaches which can be used to create digital twins of the human body to deliver a new generation of truly personalised and predictive medicine. An iterative cycle is used in Big AI – the synergy of ML and PB, where AI hypotheses are tested in physics-based simulations, and the results of PB modelling are used to train AI. A workflow has been constructed for Big AI with ensemble approaches, which overcomes the issue of variability in predictions and leads to true, and commonly non-Gaussian, statistics. Big AI could be used in many ways: some examples including tracking the spread of the virus in pandemics, predicting accurate drug binding affinities to their target proteins, accelerating drug discovery and vaccine design, and proposing drugs or drug combinations for the treatment of diseases.
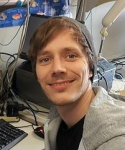
Universität Rostock & Technische Universität Berlin
WEB
Thomas Engels studied at Aachen University (Aachen, Germany), Université de Provence (Marseille, France) and Technical University Berlin (Berlin, Germany). He obtained his Ph.D. in 2015 in a joint French-German program from Aix-Marseille Université and the Technical University Berlin. The topic of his dissertation was the numerical modeling of insects in turbulence. Afterwards, he joined École Normale Supérieure (Paris, France), before becoming a postdoctoral fellow at the University of Rostock. In Paris, he developed numerical tools based on wavelets, which he combined with experiments with living animals in Rostock. He is currently affiliated with both University of Rostock and the Technical University Berlin. His interdisciplinary research combines numerical simulation, high-performance computing as well as experimental biology with living animals.
Flying insects, spectacular little flying machines with enormous evolutionary success, are an important source of inspiration for a large, interdisciplinary community of scientists. The aerodynamic mechanisms they use for propulsion are quite different from human-designed fliers, and many aspects of their locomotion are not yet understood.
In this lecture, I will illustrate what insights numerical simulations can contribute to our knowledge on the aerodynamics of flapping flight and discuss why such simulations are highly challenging. I present WABBIT, my open-source framework for performing such simulations. This code solves the Navier-Stokes equations on dynamically adapted grids with local refinement, and it runs on massively-parallel supercomputers. For grid adaptivity I use biorthogonal wavelets as a tool for compression and regularity detection.
I will show computational results on bumblebees flying in heavy turbulence, demonstrating that flapping flight in turbulence is limited by flight control, but not force production. Finally, I will conclude with the latest results on the peculiar flight of some of the smallest insects. They often feature bristled wings that have lost almost their entire membrane. Yet, those animals fly surprisingly well, and I discuss how numerical simulations helped understanding their aerodynamics.
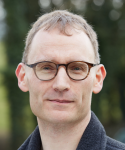
Imperial College London, United Kingdom
WEB
Neil Ferguson founded and directs the Jameel Institute and the MRC Centre for Global Infectious Disease Analysis at Imperial College London. He also heads the NIHR Health Protection Research Unit for Modelling and Health Economics. He is an International Member of the US National Academy of Medicine and a fellow of the UK Academy of Medical Sciences.
He uses mathematical and statistical models to investigate the processes shaping infectious disease pathogenesis, evolution and transmission. In addition to basic theoretical work, Professor Ferguson has applied models to study the transmission and control of multiple infectious diseases. He was educated at Oxford University where he also worked before moving to Imperial College.
He is best known for the real-time modelling of emerging infectious disease outbreaks, having worked on the transmission dynamics and control of SARS, avian (H5N1) and pandemic (H1N1) influenza, MERS-coronavirus, Ebola, Zika and now COVID-19. His other major research interest is in vector-borne diseases – he has worked on dengue throughout his career and more recently has expanded his research to include Yellow fever, Zika and malaria.
Neil has always worked at the interface of science and policy, and advises governments, the World Health Organisation and multiple other bodies on emerging infections and infectious disease epidemiology and modelling.
Epidemiological analysis and mathematical modelling have played an influential role in informing the global response to the COVID-19 pandemic. This lecture will provide an introduction to basic concepts and approaches, distinguishing between inferential modelling used to understand past trends and forward-looking (often counterfactual) modelling used to explore different epidemiological and policy scenarios. With that lens, I will review the work leading to the early characterisation of the threat posed by COVID-19 and which informed response measures world-wide. I will then discuss the difficulties posed for countries in sustaining control later in 2020 and how modelling helped to characterise new viral variants and to map the gradual relaxation of social distancing as vaccination was rolled out in 2021. Next, I will discuss the benefits of using multiple models with differing levels of complexity to address critical questions and the technical gaps in simulations of population dynamics revealed by the pandemic. I will conclude with thoughts on research priorities and lessons learned for the application of modelling and simulation in future pandemics and to other global threats.
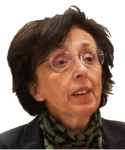
The University of Chicago, United States of America
WEB
The research activity of the Galli group focuses on the development and use and computational methods to understand and predict the behavior of solids, liquids and nanostructures from first principles. Method developments include first principles molecular dynamics, and ab initio calculations of electronic excited states, and in general electronic and vibrational spectroscopies. The theoretical studies of the interaction of matter and light focus on the prediction of optimal systems for harvesting sunlight. In addition to energy, Galli’s research covers problems related to water resources at ambient conditions and in severe environments. Other areas of interest include phenomena and materials used to realize quantum information technologies. Galli’s software activities are focused on the development of the WEST code (large-scale electronic structure within many-body perturbation theory) and participation in the development of the Qbox code (ab initio molecular dynamics) both of which are supported by MICCoM (http://miccom-center.org/).
We present theoretical and computational strategies based on quantum mechanical calculations, aimed at predicting the properties of materials and molecules with desired characteristics for quantum technologies and energy applications. We discuss computational challenges related to the development and use of interoperable codes to compute multiple properties of complex systems.
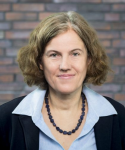
Heidelberg Institute for Theoretical Studies, Germany
WEB
Rebecca Wade studied at the University of Oxford (B.A. Hons. in physics, 1985; D. Phil. in Molecular Biophysics, 1988). She then carried out postdoctoral research at the universities of Houston and Illinois before taking up a position as a group leader in the Structural and Computational Biology Programme at the European Molecular Biology Laboratory (EMBL) in Heidelberg in 1992. Rebecca Wade set up the Molecular and Cellular Modeling Group at HITS (which was then known as the European Media Laboratory and later as EML Research) in 2001. She was Adjunct Professor at the International University in Germany in Bruchsal from 2001-2003. Rebecca Wade was appointed full Professor at Heidelberg University in 2012 and is a member of the Faculty of Biosciences. She was Scientific Director of HITS from 2015 to 2016.
Recent advances in artificial intelligence approaches to protein structure prediction offer the exciting prospect of vastly extending the number of disease targets that can be tackled by structure-based drug design approaches. Proteins are, however, constantly in motion, changing their shape and wiggling and jiggling around. Moreover, the protein motions cover a wide range of spatial and temporal scales that influence drug-target affinity and binding kinetics. The dynamic nature of protein structures thus provides challenges and opportunities for drug design. I will discuss the use of multiresolution molecular simulation techniques and machine learning to study macromolecular structural ensembles, including recent examples from studies of SARS-CoV-2 proteins.